Something is happening in the German industry. Digitization is progressing dynamically - this is the conclusion of a current study by the industry association BITKOM from May 2020. 81 percent of German companies are already using Industry 4.0 applications or plan to do so in the foreseeable future. Almost all of these organizations will change their business models in the course of Industry 4.0. On the one hand, the motor of this disruptive development is the increasing level of connectivity between industrial systems, on the other hand, AI-based technologies allow the recognition of complex patterns and correlations in huge amounts of industrial data. With the help of so-called data driven services, new business models can be developed, and the productivity of established models can be enhanced.
Based on a variety of AI projects, we present some of our most important experiences in this post. They should help you to avoid initial pitfalls in the development of data driven services.
Use Cases and Smart Services – the building blocks for new developments
What new business ideas pass the practical test? Nowadays, appropriate products or services are largely defined by customer requirements. This concerns internal customers, for example the machine operator who wants to improve the calibration process of the machine tool, as well as external customers who are interested in a pay-per-use business model, for example. At the beginning of every AI project there is the evaluation of a so-called use case - a promising application.
In use cases, in the context of industrial data analysis, information is extracted from large amounts of unstructured data and is used to generate new products and digital business models. The forms of products and business models are extremely diverse, e.g:
- Automatic detection of machine errors and subsequent requests for the most suitable service technician in case of an error,
- Calculation of the optimum machine configuration to minimize energy consumption,
- AI-supported condition monitoring of the temporal development of critical machine components.
The implementation of use cases is realized with the help of so-called Smart Services. In their core, smart services consist of analysis algorithms, that transform data in an intelligent way and so extract useful information from it. They can be categorized into technical, economic and sales-related smart services, depending on the task at hand. In sales-related Smart Services, the focus is on the usage behavior of customers. One possible use case could be the calculation of recommendations for the purchase of machine-specific consumables. In economical Smart Services, software-driven optimization of production processes is carried out, for example, with the aim of reducing failures by using the right machine configuration. The operation of the machines is monitored by technical Smart Services. They are used, for example, to detect errors in production processes or to predict the degree of wear of machine components.
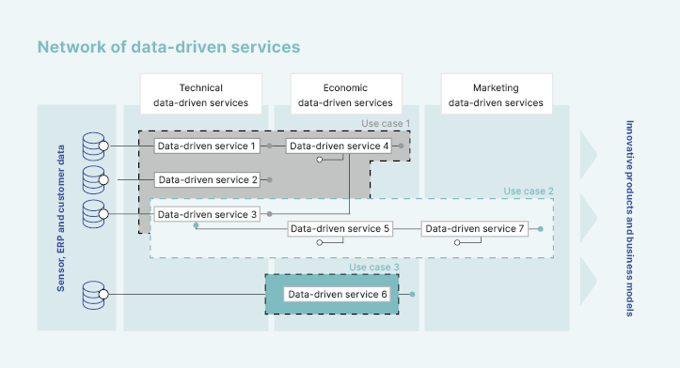
In real use cases, several networked smart services are often involved (see figure). For example, technical smart services calculate parameters for the quality status of individual machine components from sensor data, which are then aggregated by economic services (Use Case 1). By taking additional data, like e.g. machine parameters, into account, new value propositions can be created. This can include, for example, calculating the optimum machine configuration to minimize the wear of a specific component.
The conception – what distinguishes a promising use case?
The design of good use cases requires knowledge of internal processes and their weaknesses, background knowledge of customers and their needs, technical expertise, strategic knowledge and a good portion of creativity. Ideally, you will be able to develop a selection of use cases from this and evaluate their relevance for your company in a ranking.
There can be pitfalls in the technical implementation of a use case both in the implementation of the IT infrastructure and in the development of suitable analysis systems. Both are directly connected to each other. From an analysis point of view, one of the most common obstacles is an insufficient information content in the data. In order to detect this at an early stage, close coordination between business experts and data scientists is indispensable. If necessary, the data situation can be improved by appropriate measures, e.g. by using additional data sources or improved sensor technology adapted to the use case.
Use cases must be directly aligned with the customer's circumstances and requirements. If customer data play a vital role in the development of use cases, you should check whether a sufficiently large number of customers are willing to provide the necessary data. Experience shows that companies that understand and recognize the added value of data-driven approaches are also prepared to do this.
In order to develop use cases more and more efficiently in the course of corporate digitization, the smart services on which the use case is based should be intelligently modularized. Additionally, this has the positive side effect that errors are easier to identify.
The quality of the data – how to identify the data relevant for the use case
The central component of a Big Data Analytics project is data. Its quality determines the success of your use case. The concept of quality only makes sense in combination with the concrete use case and describes whether the data contains enough information in form of trends, patterns and correlations to ensure the implementation of the use case. On the one hand the amount of information is determined by the choice of data sets or measured variables and on the other hand by the measurement process itself.
For example, if you want to determine the wear condition of a bearing by vibration measurements and you position the sensor too far away from the bearing, there is a high risk that the actual wear process is only weakly detected and is additionally overlaid by other disturbing vibrations. It is therefore important that the finding an optimal measurement configuration is understood as an active scientific process in which measurement engineers and so-called technical domain experts are supported by data science know-how.
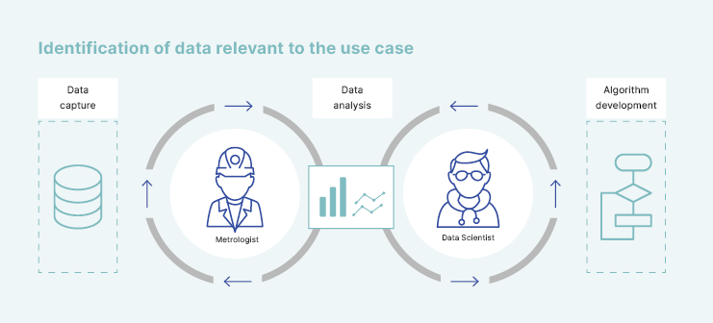
Once you have identified the data sets and measurement variables relevant for the realization of the use case, the next step is the algorithmic implementation of the solution strategy developed in the analysis phase. It is important to note that there are no universal solution formulas and algorithms to implement a complex use case. It is rather the interaction of mathematical method knowledge, domain knowledge and creativity that determines the success of the implementation.