What is customer experience, anyway? What is all the hype about? Is it just one of the many trends that happen to be in vogue at the moment, but will soon disappear into oblivion?
Customer experience is the sum of all experiences that the customer has with a company. It encompasses the subjective evaluation of the customer’s experiences at various touchpoints during a customer journey.
The customer experience management strategy attempts to make these customer experiences along the customer journey better. The ultimate concern is the connection between corporate strategy and customer experience management.
Put simply, the task of a CX strategy is to establish higher prices for products and services on the market on the basis of excellent relationships and experiences.
To do this, each employee in the company must know the following:
- what exactly the customer is to experience during contact with the company,
- what exactly justifies the higher price (and much more), and
- that the customer cannot experience these things with the competition.
- The employee must also be able to implement this added value successfully.
THE CEX trend radar: An orientation aid for decision-makers
Now, we know that it is a long journey from understanding the importance of customer experience management to implementing it. One reason for this is the many terms, methods, concepts, and technologies vying for the decision-maker’s attention and often contributing more to confusion than to orientation. For this reason, Prof. Nils Hafner of the Lucerne University of Applied Sciences and Arts and Harald Henn of Marketing Resultant developed the CEX trend radar
The CEX trend radar provides an overview of all relevant developments. It is a source of information for decision-makers that can be compiled quickly. The trend radar is based on interviews with international technology, CX, and finance experts, researchers at universities and forward-looking institutions, and public studies – and especially on in-house project experience. From the many trends, the most important technologies and instruments are prioritized according to their relevance for customer experience management.
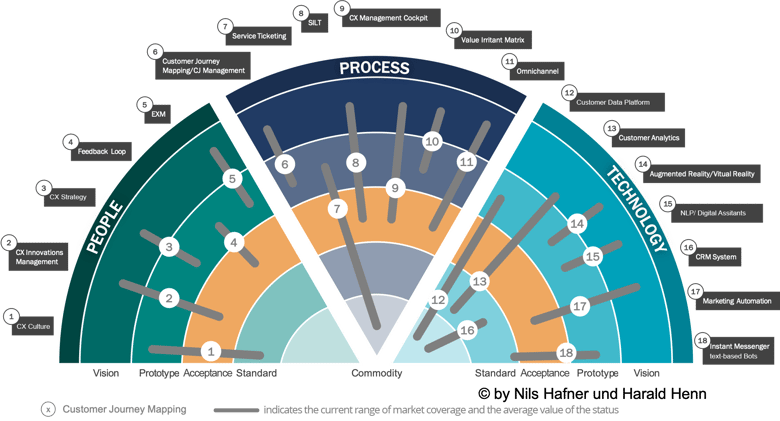
The CEX trend radar can be used to observe trends on the basis of a solid maturity model. In additional to integrating the individual issues, it estimates the maturity of each trend within each dimension. Five maturity levels (and five stages of market establishment) are laid out:
- Vision: This trend has made it onto the radar. You should keep an eye on it. But the effects on customer experience management are not sufficiently noticeable.
- Prototype: Initial efforts to implement this trend can be discerned, and companies are adopting them. Customer journey mapping applications are at this stage at the moment.
- Acceptance: This trend has established itself on the market with the companies that are the CX management leaders. But there are many implementation obstacles – and often insufficient expertise and resources. The most important trend of the last few years, marketing automation, is at this stage.
- Standard: The trend has established itself internationally. There is mature technology and widespread knowledge about its implementation and use. Recommendation: Optimize the way you capitalize on the advantages of this technology.
- Commodity: There is no longer any active conversation about this trend. Most companies are using the technology, process management technique, or employee monitoring method in question. The approach is part of CX management fundamentals. Any company that is using this foundation incompetently or not at all is suffering a competitive disadvantage.
How to use the CEX trend radar:
Each trend is numbered and assigned to one of three areas: people, process, or technology. The bar’s position shows the degree of maturity the trend has currently achieved. The figure shows the example of the “CX management cockpit” in the Vision, Prototype, and Acceptance maturity levels. The circle with the numbers is a sort of slide control. It is positioned where the majority of companies currently are. Since the trend report is completed for various industries, this control can be in different places for different industries.
Customer Experience Management in a specific example: Customer Journey Management
Let’s take a look at customer experience management using the specific issues of customer journey management and customer journey mapping. We will start with a definition. As with many terms used in conjunction with the issue of customer experience, we see a wide variety of definitions and interpretations in different publications. We understand customer journey management to be the following:
Understanding, analyzing, designing, managing, and optimizing persona-specific end-to-end customer journeys across all media and departments.
Customer journey mapping is the visualization of customer journeys (actual and target journey) from the perspective and preferences of the customer (persona-specific). In this context, user experience and customer experience are often used synonymously. We understand customer experience to be the entire experience of the customer journey. The user experience is the experience at a single touchpoint. The customer journey, not the individual touchpoint, is the relevant factor in successful differentiation on the market.
The number of touchpoints (channels customers use to communicate their concerns) is growing. Websites, apps, evaluation forums, telephone calls, and e-mails are used according to their availability and expediency. This “channel-hopping” on the part of the customer requires customer interactions to be orchestrated (customer journey orchestration), since contacts through the individual channels often occur in an uncoordinated manner within the company. Fragmented responsibility and poor documentation and storage of contact results lead to inconsistent statements, irritating the customer. Sales, marketing, and customer service are different departments within the company and thus pursue different goals. A holistic process responsibility for customer journeys (end to end) across departments with marketing and sales as internal suppliers and service providers has so far been very rare within companies, as have the associated control instruments, key indicators, and remuneration systems. Such process responsibility means a paradigm change in the organizational structure that will take a long time to complete. In practice, we observe the optimization of snippets and fragmented sections and a customer journey that falls within the responsibility of a single department. Onboarding (supporting a new customer in the initial phase of a customer relationship) and acquiring new customers in online commerce are two typical examples.
Customer Journey Mapping: Key elements
Customer journey mapping is successful and perfect when you are able to share the results with the customer and talk about them without any problem. Put another way, customer journey mapping serves less to optimize internal processes in the traditional sense than to optimize the experience from the customer’s perspective.
Internally, customer journey mapping provides a simple, self-explanatory way to show all those involved across departments what steps must be taken to optimize the customer journey.
There are no standards in customer journey mapping. Each company can (and must) decide for itself how it wishes to design its visualization, which may be detailed or rudimentary. Cosmetics are not the decisive factor. But certain ingredients are obligatory for good customer journey map content.
What makes for a good customer journey map in customer service?
Below are recommendations for issues and points that a customer journey map in customer service should contain:
- Stakeholder map. Who works with the customer, directly or indirectly? Listing all stakeholders improves understanding of performance complexity.
- Personas. Personas are fictitious individuals who represent target groups. They are a good way to see things from the customer’s perspective.
- Result. What exactly is the result the customer wants? What does he or she hope to achieve? The more concretely the expected result is identified and described, the more specifically individual touchpoints can be optimized.
- Customer Journey. Customer journey mapping in customer service means listing each individual customer step. The sequence of steps may vary from persona to persona.
- Touchpoints. Touchpoints are concrete, specific contact points between the company and the customer. An example is a specific form on a website.
- The moment of truth. Where at what touchpoints via what channels does the customer feel satisfaction or frustration?
- Responsibility/systems. For each touchpoint, the person responsible for the customer experience (the provision of a service) is noted.
- Emotional development curve along the customer journey. The only concern here is the customer’s emotional response at a touchpoint.
- Optimization potential. During visualization, participants often discover in the current journey approaches for optimization.
Customer journey mapping: Starting point for optimizing the customer experience management strategy
Experience has shown that customer journey mapping generates many approaches to optimization. In conjunction with the corporate strategy, ideas from the customer journey mapping workshop can be converted to specific target measures. This provides a binding framework for action that will produce effective customer experience management oriented on customer expectations.